Medical Diagnoses: Doctors vs. AI Accuracy
September 19, 2024 | AI vs Human
Figure out whether AI in healthcare can outperform physicians in diagnostic accuracy using machine learning and medical imaging technologies.
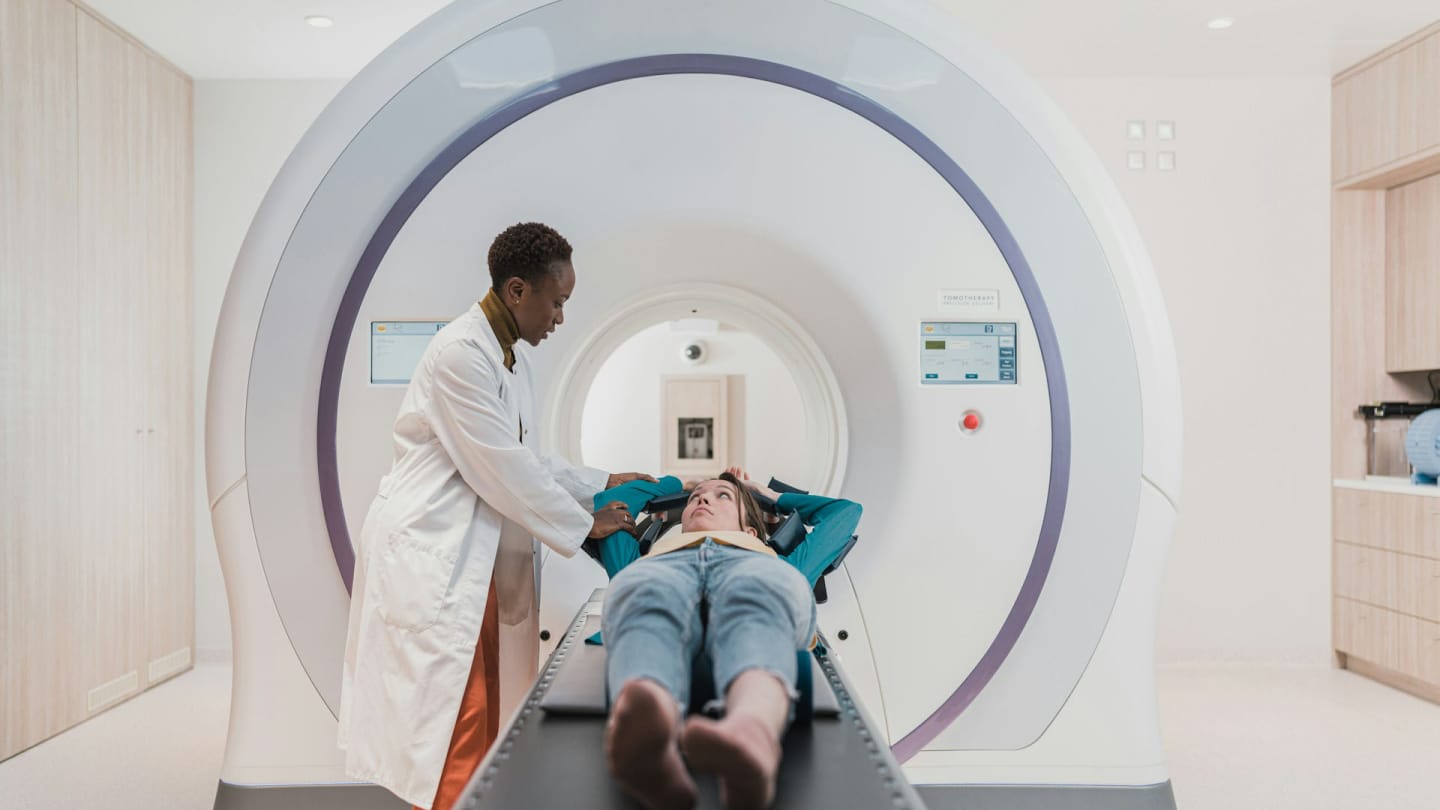
Artificial Intelligence (AI) is making waves in healthcare. It’s not just a buzzword anymore; it’s a reality transforming the way we diagnose diseases.
With advancements in machine learning and medical imaging, AI in healthcare is becoming a powerful tool.
But can AI algorithms really outperform doctors in diagnostic accuracy? This question is stirring debates among medical professionals and tech enthusiasts alike.
Understanding AI in Healthcare
Before diving into the comparison, let’s understand how AI is applied in healthcare.
What Is AI in Healthcare?
AI in healthcare involves using algorithms and software to mimic human cognition in analyzing complex medical data.
The primary aim is to improve patient outcomes by assisting doctors in diagnosis and treatment planning.
Machine Learning and Medical Imaging
In medical imaging, machine learning algorithms analyze images to detect patterns and anomalies.
- Medical Imaging Technologies: Includes X-rays, MRI scans, CT scans, and ultrasound images.
- Diagnostic Tools: AI algorithms can identify tumors, fractures, and other conditions from images.
Clinical Decision Support Systems
AI-powered clinical decision support systems (CDSS) provide healthcare professionals with data-driven insights.
- Function: Analyze patient data to suggest diagnoses or treatments.
- Benefit: Helps doctors make informed decisions quickly.
The Rise of AI Diagnostic Tools
AI diagnostic tools are becoming more prevalent. They offer several advantages over traditional methods.
Speed and Efficiency
- Rapid Analysis: AI can process vast amounts of data in seconds.
- Time-Saving: Reduces the time doctors spend on routine tasks.
Consistency
- Standardized Evaluations: AI provides consistent results without fatigue.
- Reduced Errors: Minimizes human errors caused by oversight or fatigue.
Access to Care
- Remote Diagnostics: AI tools can be used in remote areas with limited access to specialists.
- Telemedicine: Supports virtual consultations and diagnoses.
Comparing Diagnostic Accuracy
Now, let’s figure out how AI algorithms stack up against physicians in terms of diagnostic accuracy.
Studies and Real-World Examples
1. Skin Cancer Detection
A study published in Nature showed that an AI algorithm could detect skin cancer with accuracy comparable to dermatologists.
- Method: The AI was trained on thousands of skin images.
- Result: Matched or outperformed human experts in identifying cancerous lesions.
2. Retinal Disease Diagnosis
Google’s DeepMind developed an AI system to diagnose retinal diseases.
- Method: Analyzed 3D retinal scans.
- Result: Achieved over 94% accuracy, matching leading ophthalmologists.
3. Radiology and Imaging
AI algorithms have been used to detect lung nodules in CT scans.
- Benefit: Early detection of lung cancer.
- Performance: Some AI systems have shown higher sensitivity than radiologists.
Factors Influencing Accuracy
Data Quality
- Training Data: AI accuracy depends on the quality and diversity of data used for training.
- Bias: Limited or biased data can affect performance.
Complexity of Cases
- Simple vs. Complex Cases: AI performs well in straightforward cases but may struggle with complex conditions.
- Comorbidities: Multiple co-existing conditions can complicate diagnoses.
Human Expertise
- Experience: Doctors bring years of experience and intuition.
- Holistic View: Physicians consider patient history and context.
Practical Applications and Implications
AI is not just a competitor but a collaborator in healthcare.
Enhancing Physician Capabilities
- Augmented Intelligence: AI assists doctors rather than replacing them.
- Decision Support: Provides additional insights for complex cases.
Workflow Optimization
- Efficiency: Automates routine tasks, freeing up time for patient care.
- Prioritization: Helps triage patients based on urgency.
Personalized Medicine
- Tailored Treatments: AI can analyze genetic data for personalized therapies.
- Predictive Analytics: Anticipates health issues before they become severe.
Best Practices for Integrating AI in Healthcare
To maximize the benefits of AI, certain strategies should be adopted.
Collaborative Approach
- Teamwork: Encourage collaboration between AI developers and healthcare professionals.
- Training: Educate medical staff on AI tools and their applications.
Data Management
- Quality Data: Use diverse and comprehensive datasets for training.
- Privacy Compliance: Ensure patient data is protected according to regulations.
Validation and Regulation
- Clinical Trials: Test AI tools thoroughly before implementation.
- Regulatory Approval: Obtain necessary certifications from health authorities.
Ethical Considerations
- Transparency : Be open about how AI makes decisions.
- Bias Mitigation: Actively work to eliminate biases in AI algorithms.
Challenges and Limitations
Despite the potential, there are challenges to consider.
Lack of Human Touch
- Empathy: AI cannot replicate the empathy and rapport of a human doctor.
- Patient Comfort: Some patients may prefer human interaction.
Ethical and Legal Issues
- Accountability: Determining liability in case of misdiagnosis.
- Informed Consent: Patients must be aware when AI is used in their care.
Technical Limitations
- Data Quality: Incomplete or poor-quality data can lead to errors.
- Interoperability: Integrating AI systems with existing healthcare IT infrastructure.
Resistance to Change
- Adoption: Some healthcare professionals may be hesitant to adopt new technologies.
- Trust: Building trust in AI systems takes time.
AI in healthcare is a game-changer. It offers tools that can enhance diagnostic accuracy, streamline workflows, and support clinical decisions.
In certain tasks, especially those involving pattern recognition in medical imaging, AI has matched or even exceeded human accuracy.
However, AI lacks the holistic understanding, empathy, and ethical judgment that physicians bring to patient care.
The future of healthcare likely lies in collaboration between AI and human doctors.