Reducing AI Bias: Legal and Ethical Views
September 10, 2024 | Laws of AI
Understand the legal and ethical views in reducing AI bias, focusing on fairness, discrimination laws, and algorithmic accountability.
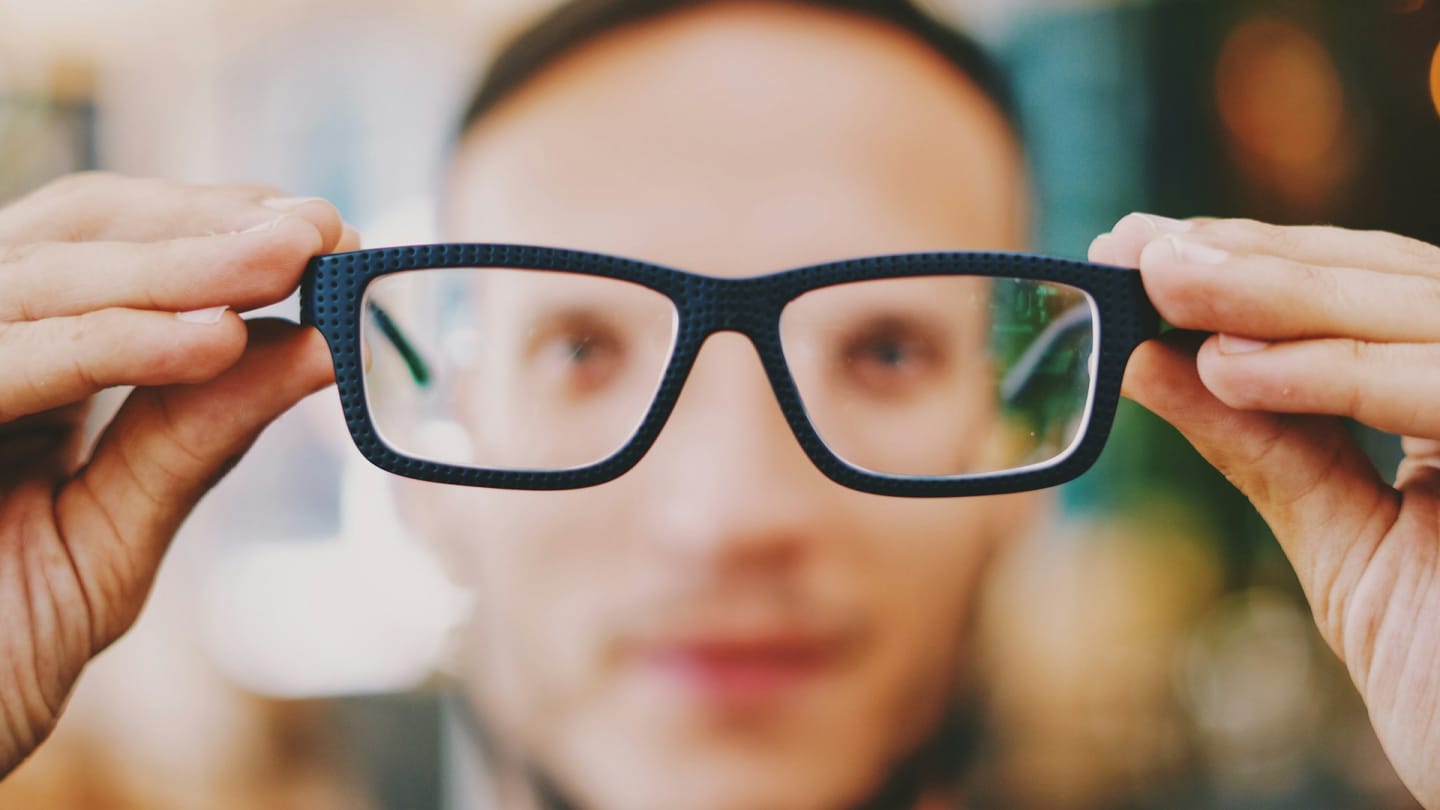
While AI holds immense potential, it also brings forth challenges, particularly concerning fairness and bias.
Reducing AI bias isn’t just a technical issue; it’s a legal and ethical imperative. AI bias can lead to unfair outcomes, affecting individuals and groups.
Explore the legal and ethical views in tackling AI bias, focusing on fairness, discrimination laws, and algorithmic accountability.
Understanding AI Bias
AI bias occurs when an AI system produces results that are systematically prejudiced due to erroneous assumptions in the machine learning process.
Types of AI Bias
- Data Bias: Arises when the data used to train the model is unrepresentative or contains historical biases.
- Algorithmic Bias: Occurs when the algorithm’s design favors certain outcomes over others.
- Interaction Bias: Emerges during the AI system’s interaction with users, reinforcing existing biases.
Real-World Examples
- Facial Recognition Systems: Some systems have higher error rates for people of color due to biased training data.
- Hiring Algorithms: AI tools may favor male candidates if trained on data from industries with gender imbalances.
Legal Views
Understanding discrimination laws and regulations is crucial for organizations deploying AI systems.
Discrimination Laws
- Equal Opportunity Laws: Prohibit discrimination based on protected characteristics like race, gender, and age.
- GDPR (General Data Protection Regulation): In the EU, GDPR mandates transparency in automated decision-making and protects individual rights.
Regulatory Compliance
- Accountability: Organizations are legally accountable for the decisions made by their AI systems.
- Transparency Requirements: Laws may require organizations to explain how their AI makes decisions.
Consequences of Non-Compliance
- Legal Penalties: Fines and sanctions for violating discrimination laws.
- Reputation Damage: Loss of public trust can have long-term impacts.
Ethical Views
Beyond legal obligations, ethical AI practices are essential for fairness and social responsibility.
Fairness and Equality
- Inclusive Design: Developing AI systems that are fair and unbiased.
- Equity vs. Equality: Ensuring equitable outcomes may require treating individuals differently based on their circumstances.
Algorithmic Accountability
- Responsibility: Developers must take responsibility for their AI systems’ outcomes.
- Transparency: Making AI decision-making processes understandable to stakeholders.
Ethical Frameworks
- Codes of Ethics: Adopting guidelines like the IEEE’s Ethically Aligned Design.
- Ethics Committees: Establishing oversight bodies within organizations.
Reducing AI Bias: Strategies and Best Practices
Implementing effective strategies can mitigate AI bias and promote fairness.
Diverse and Representative Data
- Data Auditing: Regularly assess training data for biases.
- Inclusive Data Collection: Ensure datasets represent all user groups.
Algorithmic Fairness Techniques
- Bias Mitigation Algorithms: Use techniques designed to reduce bias in AI models.
- Regular Testing: Continuously evaluate AI systems for biased outcomes.
Transparency and Explainability
- Explainable AI (XAI): Develop AI models whose decisions can be easily understood.
- Documentation: Keep detailed records of AI development processes.
Stakeholder Engagement
- User Feedback: Incorporate feedback from diverse user groups.
- Interdisciplinary Collaboration: Work with ethicists, legal experts, and sociologists.
Challenges in Reducing AI Bias
Overcoming AI bias involves navigating several challenges.
Complexity of AI Systems
- Black Box Models: Complex models like deep learning can be difficult to interpret.
- Technical Limitations: Current tools may not fully eliminate bias.
Resource Constraints
- Cost: Implementing bias mitigation strategies can be expensive.
- Expertise: Lack of skilled professionals in ethical AI.
Balancing Competing Interests
- Accuracy vs. Fairness: Improving fairness may impact model performance.
- Privacy Concerns: Transparency efforts must respect user privacy.
Legal Frameworks and Regulations
Understanding and complying with legal frameworks is essential.
Global Regulations
- EU AI Act : Proposes strict regulations on high-risk AI systems.
- Algorithmic Accountability Act (US): Aims to require impact assessments for AI systems.
Industry Standards
- ISO Standards: International standards for AI development and deployment.
- Ethical Guidelines: Industry-specific guidelines promoting ethical AI use.
Ethical AI in Practice: Case Studies
COMPAS Recidivism Algorithm
- Issue: Found to be biased against African-American defendants.
- Outcome: Sparked debates on AI fairness in the justice system.
Amazon’s Hiring Tool
- Issue: The AI favored male candidates over females.
- Action Taken: Amazon discontinued the use of the tool.
Best Practices for Organizations
Implementing best practices can help organizations address AI bias effectively.
Conduct Regular Audits
- Bias Audits: Regularly check AI systems for biased outcomes.
- Third-Party Assessments: Use independent experts for unbiased evaluations.
Develop Ethical Guidelines
- Internal Policies: Create clear policies on ethical AI use.
- Employee Training: Educate staff on ethical views.
Foster a Diverse Team
- Inclusive Hiring: Build teams with diverse backgrounds.
- Collaborative Environment: Encourage open discussions on ethics.
Future Directions
Looking ahead, ongoing efforts aim to enhance fairness and accountability in AI.
Technological Advancements
- Improved Algorithms: Developing models that inherently reduce bias.
- Explainability Tools: Advancing tools that make AI decisions transparent.
Regulatory Evolution
- Stricter Laws: Governments may implement more stringent AI regulations.
- International Cooperation: Global efforts to standardize ethical AI practices.
Reducing AI bias is crucial for ensuring fairness and upholding legal and ethical standards. Companies must identify and mitigate biases in their AI systems.
By understanding the laws, embracing ethical views, and implementing best practices, we can work towards AI systems that are fair, transparent, and accountable.
The journey towards ethical AI is continuous, requiring collaboration across disciplines and ongoing commitment.